This is a long-form follow up to #20. 🐳
One of the more absurd ways that algorithms create inequity is through digital rewards programs.
Here’s the deal: we all used to get the same set of coupons in the mail [reader: coupons still exist]. While these traditional newsprints may have been location-specific, they didn’t discriminate based on age, gender, marital or socioeconomic status — they were “dumb,” meaning they weren’t data-driven. Now, technology is causing us to lose that universality in favour of smarter, “personalized” offers that are designed to manipulate our consumption by offering different discounts based on the information we share.
The most traditional and familiar version of a loyalty program is volume-based. This scheme privileges patronage to establish and maintain brand loyalty. Think of Zeller’s now-defunct “Club Z,” Toronto-based Ritual, The Bay’s HBC Rewards, Cineplex’s Scene Points, or getting your tenth coffee free at your neighbourhood coffee shop (yum). Typically a user of such a program is rewarded with aggregating “points” that translate into a modest discount or some sort of perk. This basic exchange manifests in a range of cash rewards apps like Caddle or Swagbucks.

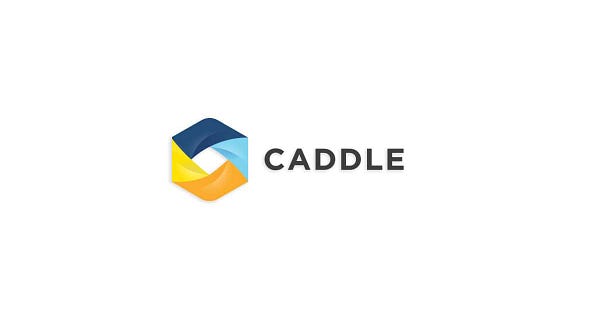
Today, many of the most lucrative and popular rewards programs tempt us from our smartphones, nudging us with personalized “offers” that are informed by our past shopping behaviour and refined by using location services to look at our movement. Earlier this year, former National Post columnist James McLeod demonstrated this trend, describing in detail how the Tim Horton’s app was logging his location in Double-Double Tracking. As a result of his documentation, Tim Horton’s is now under investigation by Canada’s Privacy Commissioner.
Not exactly the reward they were looking for.
The gamification and super-personal tailoring of these programs is new. They also create and entrench massive competitive advantages for the firms that oversee the data collection and benefit from it.
A similar example of a deeply data-driven points app is Starbucks Stars, which is volume-based but also offers “personalized” rewards and defaults to constantly collecting location information. The fine print reveals that Starbucks will offer “special” offers “customized based on your purchase behaviours.” ☕😮
Is it fair for one coffee drinker to receive a better or different offer than another just because they shop somewhere more frequently?
A distinctly 🇨🇦 Canadian example is PC Optimum - earned through shopping at Shoppers Drug Mart, PharmaPrix, Real Canadian Superstore, Murale, Loblaws, No Frills, as well as through partners like Esso and Mobil gas stations. The PC Optimum program tailors opportunities to earn points to align with the items that you buy most. While PC Optimum isn’t “Big Tech” per se, it is demonstrating a similar tactic of creating what is known as a “data moat,” which is a competitive advantage that a business holds because of its proprietary data set. For instance, the data that the apps collect on people’s movements could be used to predict and assess the viability of a new store opening or determine optimal product placement on a shelf.

These apps are quietly revolutionizing the retail ecosystem and driving their sales much more effectively than when we all received and redeemed the same offers. While simple volume-based rewards persist, the mechanism that underpins them is increasingly tailored to the individual and distinct from incentives offered to other shoppers—pushing us to purchase more, shop more often, and, most importantly, maximize expenditure. 💸
The behaviour-based ⭐“gold star” ⭐ecosystem is a powerful one (and literally its own branch of economics). It’s worth asking ourselves whether this is appropriate: who it helps, who it harms, and how. A laissez faire “why not” attitude may motivate voluntary participation in rewards regimes, but in light of economic constraints driven by COVID-19, it’s worth recognizing that in a recession, households that have lived through times of high unemployment are particularly likely to use coupons and to purchase sale items or lower-end products. This is called “scarred consumption.” So there is more urgency around this as the economy contracts—we know that more people will be driven to seek savings through these apps and other means, inadvertently making themselves vulnerable to deceptions that cannot be understood or appreciated - only redeemed.
There is also an obvious novelty to all this, prompted by big data and analytic insights. I don’t believe that companies set out with the intention of creating inequities through their reward programs. They are driven by profit maximization, and any resulting inequity is an unintended consequence. Firms have the liberty of creating any “reward” scheme that they like, and people that participate in these have granted their consent.
What no one has actually consented to is a near-constant manipulation of the marketplace for basic household goods. Invitations to save money shopping (coupons) have morphed into loyalty programs (rewards).
Galen Weston recently disclosed that the top 10% of the most active PC Optimum members earn about $59 in points every month, and that last year, members redeemed $1 billion in free goods. Keep in mind that Loblaws recently lost consumer trust after admitting to participating in the price-fixing of bread for more than 14 years. It may be difficult to truly trust the conglomerate to have a fair or appropriate rewards program, and it’s impossible to make an informed decision when you don’t know where your data is going, or how it is being used to “personalize” your offers.
It just feels important for the design of these programs to be as neutral as possible. The social justice aspect of loyalty and rewards programs is deserving of our attention and scrutiny. There are also risks from the machine learning that determines these offers: a recent study found that the sorts of algorithms used by online firms to price their products might learn to implicitly cooperate with each other, keeping prices artificially high without any direct communication and without any instructions to collude.
should regs stop riches?
We have a novel opportunity for entrepreneurial regulators sensitive to the risks that come with the goading of additional expenditure to more clearly explain those risks through labeling. Whether such algorithmic behaviour should be targeted by competition policy is an open question. We know that Competition Bureau enforcement is set to target digital services and online marketing. This enforcement should expand to consider the role of consumer protections in algorithmically-driven rewards programs. Further, policy people should be considering whether there needs to be a set of standards, or if we are comfortable with the design liberties that these companies take. At the very least, we need more algorithmic transparency so that we can understand the rationale of the personalized offers. Facebook discloses why you see what ad, and consumers should know the same when they prepare to peruse Shopper’s. There is also space to designate a more thoughtful taxonomy for these kinds of programs; based on whether they do things like monitor location data, sell user data, personalize, reward based on volume, or some combination.
Perhaps definitively understanding the calculus of personalized offers “just for you” would be the greatest *reward* of all.
☝️This song is actually kind of epic and 100% worth clicking on. #ACartApart
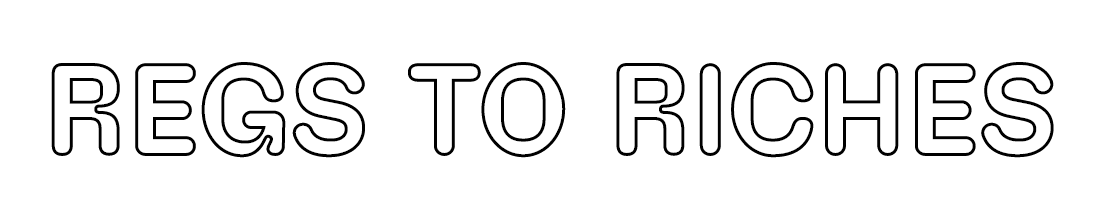